Think Cloud Services for Government, Business and Research

Cloud Market Roadmap
Cloud Service Catalogue
Tech and Market Readiness
Legal Tips
Risk Management
Cloud Standards
Final Workshop Format - FWF
Let us support you in delivering your results oriented final project event, with community, content and logistics.
Specialised Consultancy Services
Specialised content on legal aspects, risk management, cloud pricing Market and Technology Readiness Levels (MTRL).
Concertation Services
Capitalise on our consolidated community and let us help you network with likeminded projects and to maximise your visibility and improve the impact of your results..
Small Businesses
Use our tools, guides and user stories and be informed about legal, security and risk management in the cloud.
Government & Public Administrations
Easy-to-use guides and tools on procuring cloud services, managing risk, security certifications and cloud standards.
Events
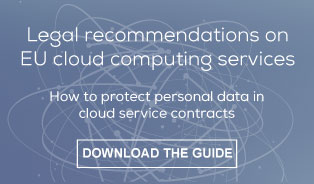
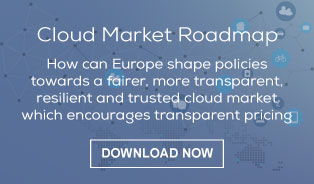
Cloud Standards Hub
Standardisation is essential for user and market confidence. CloudWATCH has developed a set of cloud standard profiles and guides to standards and security certifications, as well as organising standards plugfest events.
Cloud Research & Services
Access the EU catalogue of cloud services and see how Concertation meetings are driving clustering around cloud priorities. Find out how you too can improve exploitation activities and best plan your route to market.
CloudWATCH - Who is involved
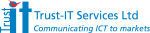




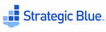